Generative artificial intelligence (GenAI) is, unquestionably, sweeping the legal profession to the brink of unprecedented technological and regulatory transformation.
According to a 2024 poll conducted by DISCO and The Cowen Group, more than 80% of legal professionals believe that document review would be heavily and immediately impacted by the introduction of GenAI into legal processes.
The good news is – while the benefits of adopting this technology are potentially profound, the learning curve for you, the legal practitioner, doesn’t need to be steep. Using generative AI for document review is about leveraging the skills you already have in a new context.
Why use generative AI for document review?
As the channels we use for communication have grown more technical and complex, so has discovery. The complexity stems not only from the proliferation of various communication methods, but also from the challenges of traditional document review.
Three key challenges of document review
- Volume and complexity
- Efficiency and cost
- Accuracy and quality
1. Volume and complexity
The sheer volume of data involved in modern litigation is overwhelming. The proliferation of digital communication platforms has only further complicated this.
Data sources include emails, text messages, social media posts, and cloud-based files, to name a few, and each source presents its own unique considerations.
The challenge lies in identifying relevant documents from these diverse data types without missing critical evidence or drowning in irrelevant data.
With GenAI, you can compress a week of review to a couple of days, providing you with a more rapid understanding of the documents in your database so you can advise your client earlier on risk and be front-footed on case strategy.
Generative AI can also do things easily that would be a huge lift for attorneys, such as provide narrative justifications for each decision. These justifications can provide attorneys with peace of mind, and — in the case of privilege — can be dropped into a privilege log with minimal editing.
Helpful resource: Ediscovery Expanded: Mastering Complex Data from Slack to Signal and Beyond
2. Efficiency and cost
Discovery is a time-intensive process, especially when dealing with large datasets that require extensive manual review. The traditional “eyes-on” approach is labor-intensive and leads to significant expenses.
Leveraging AI can help mitigate the time and cost associated with this phase of litigation, allowing you to fast-track your first-pass document review and reallocate your team’s time and budget towards more valuable strategic thinking and case-building.
3. Accuracy and quality
Accuracy is fundamental to a successful document review – but human error and subjectivity present significant challenges to maintaining consistent standards across multiple reviewers.
Leveraging LLMs for document review creates a partnership between human reviewers and GenAI tools, allowing a small team to identify relevant documents more accurately and reduce the risk of overlooking important information.
Example: Generative AI-powered document review in action
Samika, a junior associate, is working on a case with over 100,000 documents. Rather than tagging documents to provide training examples for an AI model (or, heaven forbid, reviewing documents one by one herself), she can leverage large language models and generative AI.
How? Samika can write the same kind of document review protocol she would write during any other review, and simply give the context and definitions for each tag to a tool powered by generative AI.
For instance, she can tell the tool that “privileged” means:
“Any communication between a lawyer and their client discussing legal matters.”
The tool will then read each document, decide whether the document meets that criterion, and tag the document accordingly. Using generative AI, the tool can also provide a natural language justification, such as:
“This document is an email between Attorney X and Client Y discussing whether they should accept revisions to a contract.”
If Samika is using a tool like DISCO’s Cecilia Auto Review, her entire review can be completed in a week – even if she is the only person on the team.
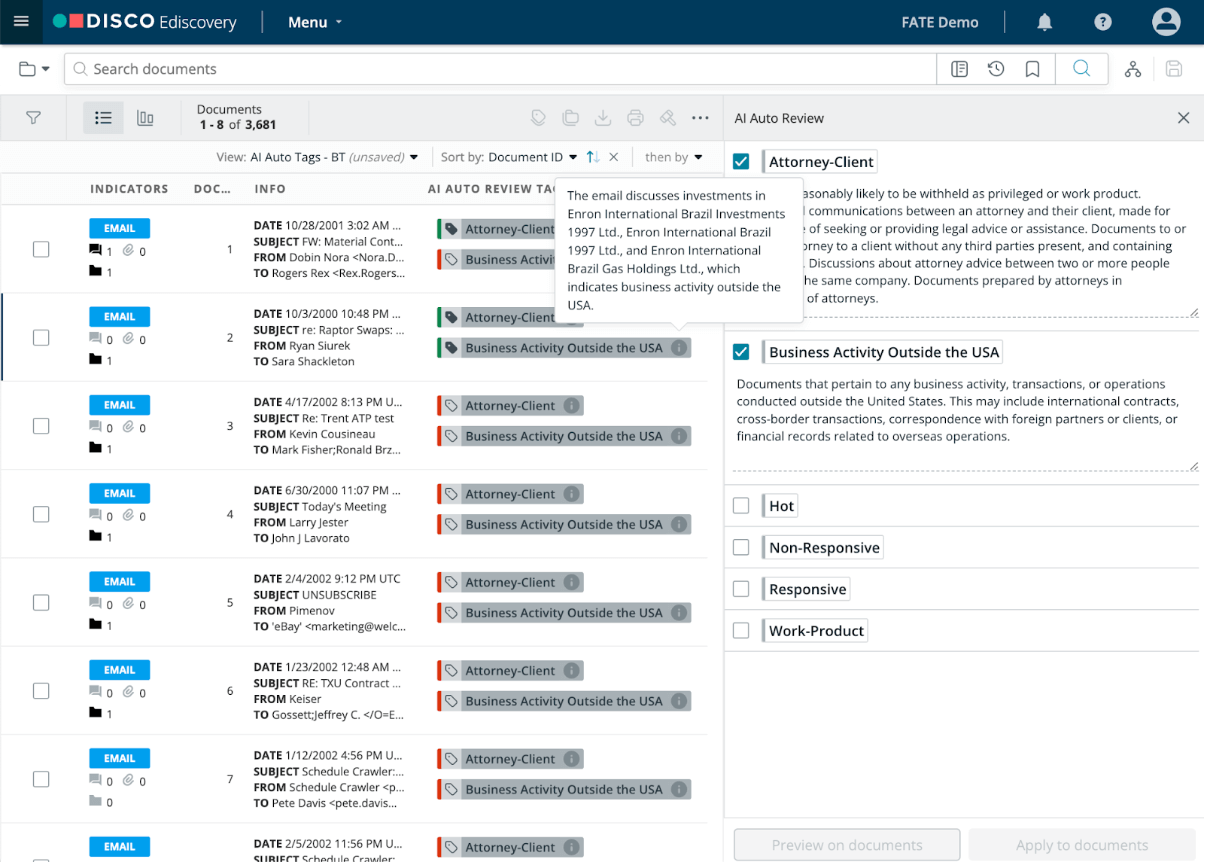
Ethics and compliance in generative AI-powered document review
Legal professionals are required to adhere to strict ethical standards, which include supervising non-lawyer employees, and retaining all responsibility for the legal work done on behalf of clients. Maintaining data privacy is also a paramount concern, so be sure to choose an AI provider that prioritizes security and compliance
The good news is, technology-assisted legal workflows have long been approved by courts, notably in Da Silva Moore v. Publicis Groupe, 287 F.R.D. 182 (S.D.N.Y. 2012). Generative AI may be novel, but it follows a long precedent of AI being regarded as an accepted – even integral – element of good legal representation.
Additionally, make sure any AI tool you use for ediscovery is designed to comply with relevant data protection regulations. Hosted on AWS global infrastructure, DISCO’s tech upholds the highest standards for privacy and data security. This level of compliance, including SOC2 Type 2 and ISO 27001 certification, as well as GDPR and HIPAA compliance, ensures that sensitive client data is protected at all times.
Related: Case study: DISCO Review Shines with High Accuracy and Increased Efficiencies 💡
How GenAI addresses the challenges of contemporary document review
To effectively use GenAI – for document review or any workflow – you have to understand a bit about how it works.
Large language models (LLMs)
Large language models (LLMs) are machine learning models that can process and generate text in human language. This creates the powerful (though not quite accurate) impression that the model is able to understand your questions.
GenAI uses LLMs to generate new content based on the data sets they were trained on. These include tools like ChatGPT, Google Gemini, Jasper, and Claude.
Responses generated by GenAI can be very useful – but note that LLMs do not actually “understand your question” in the way you’d expect a human listener or reader to do. Rather, they are processing an incredible amount of data – the “large” in “large language model” – to calculate probabilities and construct an answer that has the highest likelihood of being realistic.
Essentially, LLMs are the world’s smartest auto-complete, however, they are the best type of AI for document review.
What GenAI and LLMs mean for document review
So, what does this mean for document review? And how can legal practitioners prepare for the world in which this technology is commonly used for this workflow?
The good news is, litigators are already experts in crafting review protocols and leading teams of human reviewers through first-pass doc review. To a large extent, effectively using GenAI for document review means adapting these existing skillsets, taking into account the strengths, limitations, and operational differences of working with LLMs.
Setting up your first GenAI-powered document review
When you set up a review with humans, you design a document review protocol that serves to define the scope for when a tag should or shouldn’t apply. The process for starting a document review project with GenAI starts the same way.
The descriptions in your review protocol are the foundation and initial source of the tag descriptions you’d input into a GenAI tool. A few key differences are:
- Tools like DISCO’s GenAI doc review tool, Cecilia Auto Review, predict tags based on text within the four corners of documents only. Tag descriptions should not be based on metadata.
- Tags operate independently – so each tag description should stand on its own rather than rely on other tags.
- Tags should refer to the content of a document (e.g. responsive, non-responsive, hot, key, important) rather than the workflows (e.g., further review, tech defect, ready for production etc.)
- Tag instructions should be clear and concise.
When creating tagging descriptions, it is helpful to gather the relevant documentation (RFPs, review protocol, etc.) and map the relevant paragraphs of those documents to the particular tag you’re writing a description for, which will ensure that the scope is fully covered with the tag descriptions.
Putting the mapping exercise into action
First, list all of the relevant language from the documentation. Assign each to the correct tag.
e.g.,
Once this is complete, add all of the relevant documentation paragraphs to each tag description table. Generate the tag description based on the language from the source.
e.g.,
These are the tag descriptions you would then test on your alignment sample (see “Quality Control”) or, ultimately, in your LLM-guided review.
Remember: No one is better at wordsmithing than attorneys, and guiding LLMs for document review requires being clear and patient, and realizing that you might have to ask things in a different way to achieve what you want.
So take a deep breath, relax, and enjoy using GenAI for first-pass document review to achieve much faster, more accurate, and more consistent results. In the next section, we’ll cover 7 best practices for writing tag descriptions for GenAI that return the optimal results.
How to write tag descriptions for GenAI-guided document review: 7 best practices
Building on the understanding of the differences between human brains and LLMs we outlined above, DISCO’s AI and document review experts recommend the following for successfully writing tag descriptions for AI-led review:
1. Be extremely explicit
As with humans, so with LLMs: The more explicit you can be, the better. Focus on the task of outlining clear criteria for each tag, e.g., “Documents that contain X.”
Pro tips for avoiding ambiguity:
- Define unusual and technical terms, or terms that are used in ways laypeople ordinarily wouldn’t.
- Ensure your pronouns have clear antecedents. (e.g., the identity of “she” in the sentence, “Sally and Ana worked together until she was terminated,” is unclear and confusing.)
- Use first and last names. When possible, also include aliases and email addresses.
For example, imagine you’re looking for documents showing fraud and want to provide your GenAI tool with a tag description:
- Bad tag description: Documents showing that the defendants committed fraud.
- Good tag description: Documents showing that the Defendants, defined as Company A or its employees, including John Smith or Jane Smith, committed fraud. This includes documents showing that Defendants misrepresented the abilities of their tooth whitening product, Product Name, including by forging documents and studies. Any documents showing fraud or deceptive behavior by Defendants related to Product Name are relevant. This does not include news articles concerning election fraud.
Per DISCO’s Senior Director of Machine Learning and Artificial Intelligence, Dr. Robert Harrington, “It’s a matter of being clear in what you’re asking the LLM to do, and being patient. And who’s better at this than attorneys?”
2. Write at an 8th-grade level
…and to the extent you can, avoid archaic or extremely obscure words.The reason? LLMs are trained on enormous language sets – meaning, the entire internet. Generally speaking, most web copy is written at an 8th-grade level. This means most LLMs you interact with “understand,” or operate best, in 8th-grade-level language.
DISCO's Director of Product Management notes, “We’ve done some tweaking to get our LLM (underlying Cecilia Auto Review) to be more legally oriented – but there’s not enough legal language out there to train a powerful large language model all on its own. Even if you fed it the entire corpus of US case law, it wouldn’t be enough. What this means is, the vast majority of text fed into almost any LLM is people speaking normally – Facebook posts, Twitter posts, news articles."
Don’t be afraid to use words that are in the standard lexicon – most LLMs will know, for example, what a subpoena is. But for the large part, you’ll have more success with less sophisticated language.
3. Carefully consider the order of information
If you’re consistently not getting the results you want from a GenAI prompt – especially a lengthy one with a lot of information – try changing the order.
LLMs are subject to recency bias – and secondarily, to primacy bias. This means they pay the most attention to what comes last in a prompt, and some attention to what comes first. The bits in the middle tend to get lost.Why? LLMs are trained on the way normal people speak and write – and humans tend to put the most important information in introductions (context-setting) and conclusions (instructions and takeaways). This is relevant even at the level of individual tag descriptions.
Per DISCO's Director of Product Management, “Contextual stuff is a problem in general – you need to tell the LLM what it’s meant to be doing up front before it processes the content. But because of recency bias, it’s also best to reiterate it again at the end.”
The tl;dr: Put the most important information at the end of your prompt.
4. Stay within the four corners of the document
This is one of the key differences between doing document review with humans and with GenAI.
Humans are able to gather much greater context during doc review, such as the custodians of each document. In contrast, in the case of DISCO’s Cecilia Auto Review, the LLM is not reading the metadata – it’s not reading anything other than the text of the document.
5. Keep descriptions short and simple – and if you need to, break them up
Don’t write verbose paragraphs, notes James Park, DISCO Director of AI Consulting. You can be short and choppy in your writing, almost like you’re writing a bulleted list.
DISCO's Director of Product Management agrees: “Short and simple is better. There’s less room for interpretation and error.”
In fact, rather than writing a very complex tag description, you may be better served by breaking it up. Split compound tags into multiple tags – then, later, run a search that “ands” them together.
6. Test, tweak, try again
James Park notes, “With human reviewers, as they start the review, you might start getting questions from them that allow you to iterate on and refine your tag descriptions. “It’s the same with LLM – as you start getting the output, you can increase the clarity.”
(Learn more about this process in the “Quality control” section that follows.)
7. Trust your skills
If you’re reading this guide, you’re likely already familiar with the process of leading teams of human reviewers through review protocols. Despite the differences between humans and LLMs, those skills will take you most of the way there. Dr. Robert Harrington advises, “Take a deep breath and relax – attorneys are already really good at words. You’re already going to be better at this than data scientists. Whenever I’m stuck on my team on writing a good prompt, I go to an attorney in the DISCO team and ask for help.”
Quality control: How to understand if your LLM-guided review is making accurate tagging decisions
When you give humans instructions, there is variability in comprehension, which can lead to dramatic inconsistencies in how certain guidelines are applied. A team of ten human reviewers might each interpret things differently, whereas one LLM is consistent in its reviews.
This means that your quality control (QC) practices will revolve around consistency.
For example, if you’re doing QC on a set of documents reviewed by a team of human reviewers, you might find errors that are specific to a particular reviewer. This would prompt you to take a closer look at more of the documents that that particular reviewer tagged.
By contrast, if you find a mistake with how a GenAI tool reviewed a document, that mistake was likely made across all documents. In this case, you should iterate on your instructions and try again.
Additionally, when human reviewers start asking questions as they begin tagging documents, you might realize that your instructions were unclear or ambiguous. An LLM won’t ask you questions – however, as you start getting outputs and noticing discrepancies from what you wanted or expected to see, you can increase the clarity of your tag descriptions.
This is why it’s crucial to not run your full review before you validate that the AI is returning the results you want. Validating and iterating are key.
Using the best practices above, you’ve written and input your tag descriptions. Now, just like in a human-led first-pass review, it’s time to see if the AI is returning the results you want.
Use the following process to optimize your LLM-guided document review.
Run an alignment sample
⛔ Don’t create your tag descriptions and then run a review over the entire document set.
Instead, compile a sample of random documents from the population – say, 500 documents. (Do not include related documents, like family or thread members.) For optimal review hygiene, create a subfolder for this sample, e.g., “Alignment Sample 01.”
Have a small team of one to three trusted, high-quality human reviewers review the sample. Then, have Cecilia Auto Review or your GenAI-powered review tool review the same sample.
Check the AI’s results against human reviewers’
Check the human reviewers’ tagging decisions against those made by the GenAI-powered review tool.
These will fall into the following categories:
- Agreement: Both the human reviewers and Cecilia Auto Review (or your tool of choice) marked the same documents as responsive.
- Agreement: Both the human reviewers and Cecilia Auto Review (or your tool of choice) marked the same documents as not responsive.
- Disagreement: Cecilia has marked documents responsive that the human reviewers didn’t.
- Disagreement: Cecilia didn’t mark documents as responsive that human reviewers did.
Analyze the discrepancies and iterate
Carefully review the conflicts to identify themes and trends. As you go, take notes (in Cecilia Auto Review, this is in the Annotations feature or Document Note field), describing the content that makes the document responsive.
Once you’ve completed this review, examine your notes to determine any patterns in the documents that the AI missed. Based on the findings, adjust your tag descriptions accordingly.
- Too many false positives: If Cecilia Auto Review or your other GenAI doc review tool has incorrectly marked too many documents responsive, rewrite your descriptions with greater clarity around what should be tagged, perhaps with examples of what should not be tagged.
- Too many false negatives: If Cecilia Auto Review or your other GenAI doc review tool has missed too many responsive documents, rewrite your descriptions with greater clarity around what should be tagged, perhaps with examples of what should be tagged.
Then, run the sample through your AI tool again and repeat the process until you’ve reached satisfactory levels of agreement.
Run a second alignment sample
This ensures your tag descriptions aren’t overfitted – i.e., too tailored to the documents in your previous sample.
Select a different random sample of documents that excludes your original sample (again, taking care not to include family or thread members), and add them to a subfolder, e.g., “Alignment Sample 02.” Take care not to include documents from your first alignment sample!
Repeat the process outlined for the first alignment sample and make adjustments to the tag descriptions where needed.
How to validate your results
To understand the results of your review, you’ll need to understand the following terms and why they matter:
- Precision: A measure of how many of the documents retrieved are correctly categorized as responsive; i.e., what fraction of the time the AI is correct. Low precision indicates a large number of non-responsive documents incorrectly categorized as responsive.
- Recall: A measure of how many of the relevant documents have been found. Low recall means a large number of relevant or responsive documents have not been found.
What to expect when conducting Generative AI-driven document review
The process for getting a review started with GenAI is not drastically different from doing so with humans – but the results are.
Speed and consistency are the greatest benefits you’ll see from LLMs. DISCO’s Generative AI document review tool, Cecilia Auto Review, has demonstrated review speeds of 3,800 documents per hour — over a 24-hour period, equivalent to a 140-person review team working an eight-hour day — and precision and recall metrics 10-20% higher than typical from human reviewers.
It’s not unusual for a technological breakthrough to introduce some order of efficiency into your workflows. Using the best technology is a differentiator in today’s world, where clients put the highest value on proactive and forward-looking attorneys.
GenAI is not replacing legal judgment and the role of the human. Instead, it’s one powerful resource in your toolbox that allows you to improve accuracy and turnaround time for document review while automating the manual and tedious tasks.
We can’t reiterate this enough: GenAI will not replace human legal practitioners. Humans play a critical role in getting the most out of GenAI by writing clear and specific instructions, which we’ll cover in the next section.
What you need to succeed in the new landscape of AI legal document review
Tomorrow’s competitive practitioners will be able to instruct GenAI to generate faster, more accurate, and more consistent results.
Generative AI is going to irrevocably change the way legal teams perform document review, and soon. The good news is: Between the guidance above and your extant review protocol expertise, you already have the skills you need to make the leap.
That partnership between attorneys and AI formed the origins of DISCO’s AI Lab more than 10 years ago, and which has brought to market GenAI-powered legal tools like Cecilia Q&A, Depo Summaries, Doc Summaries, and Timelines – and now, Cecilia Auto Review.
Cecilia Auto Review is a new offering in DISCO Ediscovery that uses generative AI to complete first-pass document review. Simply provide Auto Review with tag descriptions, using the best practices described above, and start your review. Ready to QC? Auto Review provides explanations for each tagging decision, enabling precisely targeted quality control with no sacrifice in quality, and can complete reviews in a fraction of the time of human reviewers, cutting weeks to days or even hours.
To learn more from the experts in DISCO’s AI Lab and see a demo of Cecilia Auto Review, get in touch.