Reality Check: How to Navigate AI Hallucinations
Unless you’ve been under a rock the last two years, it’s hard to escape news about generative AI hallucination horror stories in the legal industry. And perhaps none have been more widely reported than the troubles of the attorneys in New York and Boston who relied on ChatGPT for legal research, only to learn that the judges in their respective cases couldn’t find some of the caselaw – because it didn’t exist.
The AI, in short, had hallucinated.
As generative artificial intelligence (generative AI, or GenAI) becomes increasingly integrated into our daily lives and workflows, follow these tips to spot AI hallucinations and ensure the information you receive from GenAI is reliable and trustworthy.
Contents
- Different types of AI
- What is a GenAI hallucination?
- Types of GenAI hallucinations
- What causes GenAI hallucinations?
- 10 tips to combat GenAI hallucinations
- What to look for in vetting GenAI tools

Laying the table: different types of AI
GenAI has become a bit of a catch-all term, but it’s really the latest in a series of types of AI, including a couple we’re all so familiar with in the legal and ediscovery industries we probably don’t even think of them as AI:
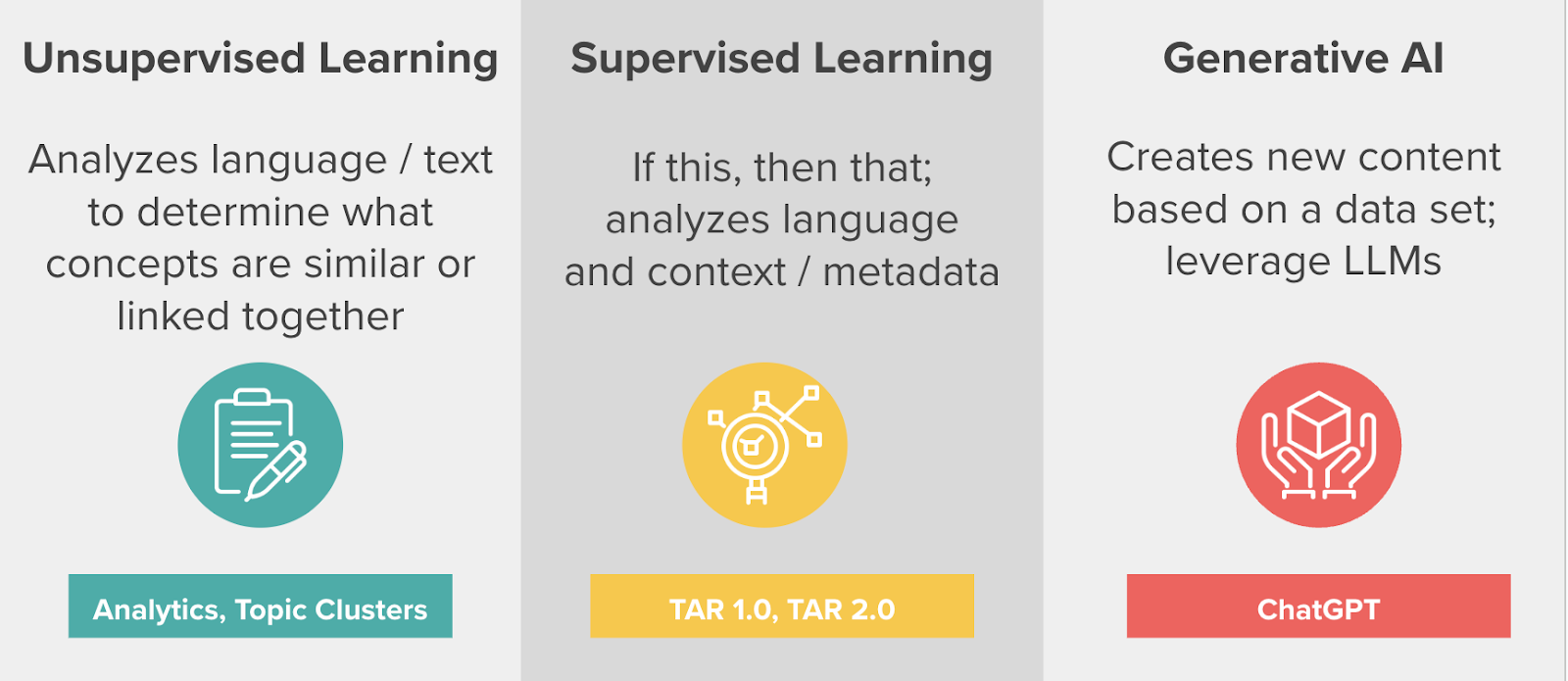
- Unsupervised learning: analyzes text to determine what concepts are similar or linked together. Seen in analytics and topic clustering functions.
- Supervised learning: analyzes context and metadata to make “if this, then that” statements. Seen in TAR 1.0 (so-called “predictive coding”) and TAR 2.0 (or continuous learning-based workflows).
- Generative AI (GenAI): creates new content based on a data set and leverages large language models (LLM). Seen in ChatGPT.
This is by no means an exhaustive list of the different kinds of AI, but for purposes of this blog post, we’ll focus on GenAI – as uniquely susceptible to the challenge of hallucinations.
What is a GenAI hallucination?
Because LLMs are designed to produce fluent, coherent text, when a tool perceives nonexistent patterns, it provides answers that can appear plausible, but might well be untrue – and even complete nonsense. These can range from minor inconsistencies to completely fabricated information, and are referred to as AI hallucinations.
The term “hallucination” was first applied to AI systems in the late 2010s, and gained wider recognition during the AI boom of the early 2020s when Meta warned that algorithms are prone to hallucinate, i.e., “confidently state information that isn’t correct.”
Types of GenAI hallucinations
There are several types of hallucinations, including the following examples.
Sentence contradiction
This is when an LLM generates a sentence that contradicts a previous sentence. For example:
Prompt: "Write a description of a landscape in four-word sentences."
Output: "The grass was green. The mountains were blue. The river was purple. The grass was brown."
Prompt contradiction
This is when the response may be technically accurate, but does not correctly answer the prompt. For example:
Prompt: "Write a birthday card for my niece."
Output: "Happy anniversary, Mom and Dad!"
Factual contradiction
This is when the AI generates fictitious information and presents it as a fact – such as the AI-hallucinated caselaw I referenced in the introduction. For example:
Prompt: "Name three cities in the United States."
Output: "New York, Los Angeles, Toronto."
Irrelevant or random hallucinations
This is when random information with no pertinence to the output or input is generated, or where a relevant piece of information is twinned with an irrelevant one.
Prompt: "Describe London to me."
Output: "London is a city in England. Cats need to be fed at least once a day."
What causes GenAI hallucinations?
GenAI uses large language models (LLMs), which are machine learning models that can process and generate text in human language – conveying the powerful (and very useful!) impression that something like a ChatGPT is able to understand your questions, and can give you a response in plain English (or another language).
Those responses are often very useful, but note that what ChatGPT is doing is not “understanding the question” in the way you’d expect a human listener or reader to do. Rather, ChatGPT is processing an incredible amount of data – the “large” in “large language model” – to calculate probabilities and construct an answer that has the highest likelihood of being true.
Conversely, precisely because LLMs make predictions by finding patterns in the data, if the training data is incomplete or biased, the model may learn incorrect patterns and generate incorrect predictions, aka, hallucinations.
Stated differently, a GenAI hallucination isn’t just wrong information – it is information that has some probability of being true (but isn’t in fact true!). And because LLMs are designed to produce fluent, coherent text, when a tool perceives nonexistent patterns, it creates outcomes that can range from minor inconsistencies to complete nonsense, but yet appear plausible.
How to prevent GenAI hallucinations: 10 tips
LLMs are not capable of determining accuracy; they only predict what ordering of words will have the highest probability of success.
As a user, there are some steps you can take to mitigate the risk of hallucinations when using GenAI tools.
1. Refine results with multiple prompts
Multiple prompts help at two levels:
First, you are able to break complex requests down into individual steps that you can fact-check at each stage, helping eliminate the opportunity for error.
Second, framing multiple prompts takes into account the nature of GenAI – as a calculator of probabilities and not a dispenser of truth. Think of this as a feature, not a bug. The process of trying to get answers should reflect that.
For example:
Prompt 1: Read the report, attached.* Please provide a 20-30 word summary of each section.
Prompt 2: Condense each summarized section into 1-2 high-level bullet points of 5-10 words each.
Prompt 3: Draft an email I can share with my boss, the CFO of a large automotive company, briefly introducing the report and containing a list of these bullet points. The language should be formal and brief.
*Did you know? With Cecilia doc summaries and other AI tools, you can upload a document, table, or PDF, and the generative AI tool will read it in seconds.
2. Be specific with your GenAI prompts
When requesting an ask from a GenAI tool, specificity is key.
Providing clear and detailed instructions can guide the AI toward generating the desired output without leaving too much room for interpretation. This means specifying context, desired details, and even citing sources.
Good prompt: Detail the diplomatic tensions that led to Napoleon’s conflict in the Battle of Waterloo.
Bad prompt: Tell me about Napoleon’s last war.
3. If you are unsure about a fact, don’t assume or guess – just ask
Guessing can lead to what we call “false premise fallacy,” i.e., the assumption of facts.
Let’s say you are unsure whether FDR or Truman was the U.S. President who attended the Potsdam conference in 1945. Rather than guessing and assuming that the LLM will correct your mistake, ask a series of questions to help get you to a better answer.
For instance:
Good prompt, part 1: Which U.S. President attended the Potsdam conference towards the end of World War II?
(Answer: President Harry Truman.)
Good prompt, part 2: What was President Truman’s position at the Potsdam conference towards the end of World War II?
Bad prompt: What was FDR’s position at the Potsdam conference?
4. Assign the AI a role, profession, or expertise
Try asking the AI to put itself in the shoes of an expert. It gives the tool more context behind the prompt and influences the style of the response.
For example:
Prompt: As a fitness coach, could you provide a plan for someone who is just starting to get fit?
Prompt: You are a lawyer. What’s the first thing I should consider when filing a patent?
Prompt: As a financial advisor, what are the best strategies for saving for retirement when you’re in your 30s?
5. Define the audience for your GenAI prompt
What happens when the answer given by the AI isn’t technically wrong – but is wrong for your use case?
Get a better answer by telling the AI which audience it’s speaking to. For example, these prompts will yield different results:
Prompt: How does photosynthesis work? Imagine you’re speaking to an intelligent 10-year-old.
Prompt: How does leaf color affect photosynthesis? Imagine you’re speaking to a group of trained scientists who already understand the basics of photosynthesis.
6. Tell the GenAI what you don’t want
Preemptively guiding an AI’s response by “negative prompting” can allow you to tell the tool what you expect to see in the response and narrow its focus, such as “don’t include data older than five years.”
Prompt: What are five themed cocktails I can serve at my beachfront wedding? Don’t include anything with gin.
7. Ask the AI to cite key info or sources
Imagine you want a list of highly rated horror movies that have come out in the past five years.
There’s a lot of room for error here. You may end up with movies on the list that were made earlier than five years ago – or that aren’t actually highly rated.
Ask the GenAI tool to include key information in the responses – as well as links to where that information came from.
Weaker prompt: “Give me a list of 20 horror movies that came out in the past 5 years and have Rotten Tomatoes scores of 90%.”
Stronger prompt: “Give me a list of 20 highly rated horror movies that have come out in the past 5 years. For each film, list the year it was released, the Rotten Tomatoes score, and a link to the review webpage.”
8. Verify, verify, verify
Despite advances in AI, human reviewers remain one of the most effective safeguards against hallucinations. Make sure to regularly review AI-generated content for errors or fabrications.
9. Provide feedback
If the AI gets the answer wrong the first time – or includes information you specifically asked to be excluded – tell it so and ask it to try again.
You can even encourage the LLM to be honest, with statements like, “If you do not know the answer, just say ‘I don’t know.’”
For example:
Prompt 1: Please provide a list of 10 books written by women in the 1940s about domestic life.
Prompt 2 (follow-up): Some of these books were published in the 1960s and some are not about domestic life. Please try again. If you can’t find 10 books matching these criteria, you can just say, ‘I can’t find 10 books matching the criteria of being written by women, about domestic life, and published in the 1940s.”
10. Use a trusted LLM
Research that the GenAI platform you’re using is built on a trusted LLM that’s as free of bias as possible, like DISCO’s Cecilia AI. A generic LLM can be useful for less sensitive tasks like drafting an email, but be sure to not input any sensitive or protected information.
What to look for when vetting GenAI tools
No technology is perfect. When considering AI tools and vendors, look for models that:
- Include specific citations
- Do not train on customer data
- Allow you to evaluate the outputs
All of DISCO’s GenAI features provide answers with sources cited, so you can use your legal judgment to evaluate the responses generated against underlying documents or depositions.
Plus, DISCO only uses LLMs that are not permitted to train on nor store customer data. That’s what built for lawyers means.
Learn more about our suite of GenAI legaltech solutions here – or, ask us directly. Request a demo.